FirstMark’s Matt Turck: ‘The Data Space Has Been the Gift That Keeps on Giving’
One of the sector’s best-known investors and community builders on the countless opportunities to come up with a better tool, better platform, and better application in data and AI.
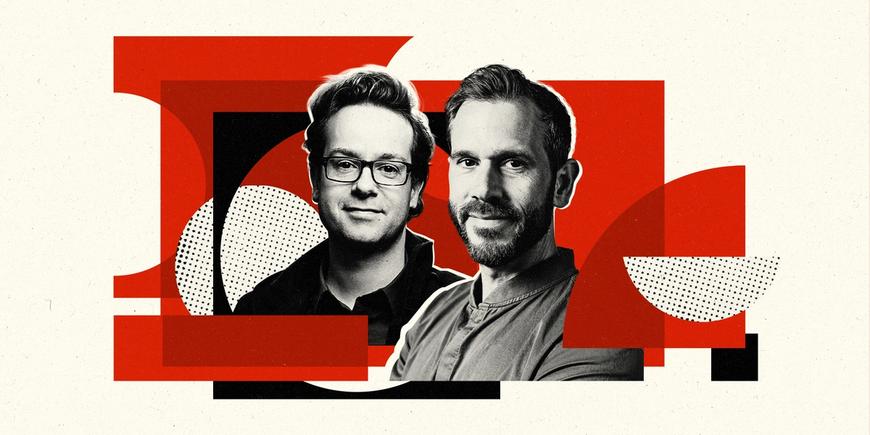
Matt Turck is Managing Director at FirstMark, where he focuses on early stage enterprise, cloud, data and ML/AI investing. Matt has always been passionate about building communities and has been running Data Driven NYC, the largest data and AI community in the U.S., since 2011, back when he was setting up Bloomberg Ventures. Prior to that, he was an AI founder himself.
Matt recently joined Primary’s NYC Founders Fellowship to discuss how he got started, why he loves building communities, the countless opportunities companies have for development and growth, and why entrepreneurs should focus on solving real customer problems with their products.
Let’s start off with the beginning of your journey. Why did you initially organize a meetup?
Community building has become a very trendy topic, but 10 years ago it was all still relatively nascent. I was originally looking for a place where people that were geeky about data topics could get together. I couldn’t find something like that, so on a Sunday night I decided on a whim, "I'll just create something” and landed on meetup.com which was basically the one option at the time. I figured I would invite 10 friends and we'd gather around the topic for an hour. Then, at the first event, we ended up with 30 people. At the second event, I thought I was going to have 30 and ended up with 60, and so on and so forth. Pretty quickly we ended up with hundreds of people trying to get a seat at the event. So, in other words, it was not planned. It was just an idea I had, that happened to meet a market need, as it turned out.
Why data? What was the interest that you had at that time?
I’ve been passionate about data and AI pretty much my entire career. A couple of years after graduating from school, I cofounded a startup that did enterprise search software and used a lot of Bayesian techniques that were the state of the art in AI at the time, as deep learning wasn’t really a thing yet. After a few years, that startup was acquired by Oracle, which I joined as part of the acquisition. At Oracle, I went from the world of unstructured data (which our startup focused on) to the world of structured data and databases. Then I left to go work at Bloomberg and helped start Bloomberg Ventures, which was the first incubation and venture effort of Bloomberg LP. There I got deeply immersed in the world of real-time, mission-critical financial data.
So basically I had been in and out of data and AI as a founder and operator my entire career up until then. So focusing on those areas as a VC was a very natural evolution, and building a community around data and AI on the side felt very organic as well.
What has surprised you in particular about the evolution of not just the community, but the data space?
The data and AI space has been the gift that keeps on giving, both for founders and investors. The term “megatrend” is overused, but it certainly applies here. It’s been playing out for a few decades already, and it’s likely to continue for a long time. The pace of innovation in that broad space has been amazing. There's one thing, and then there's a new thing, and then there's a new-new thing, and then the new-new-new thing. In data infrastructure, there’s been the evolution from on prem data warehouses to Hadoop to cloud services to Snowflake and the Modern Data Stack. In AI, there’s been this amazing acceleration from Good Old Fashioned AI to deep learning to reinforcement learning to GANs and these days, what feels like a new Large Language Model every week. If you're an entrepreneur, there are countless opportunities to come up with a better tool, better platform, and better application. There’s always something to do and build, which is fun.
I think that you have a perspective on every company becoming a data company that I’d love to hear.
The cheap analogy here is that if you’re not at least somewhat data-driven as a company, you’re flying blind. All of us have senses—see, smell, taste; that's data to the enterprise. It's the way a company can make sense of what's around it, what's in it, what was before it, what may be ahead of it, and saying that you don't care about data is sort of saying, "Oh, I'm pretty happy to be mute and blind and deaf."
Data used to be something that you would collect in small volumes through surveys and conversations, but as we all know, in the last 20 years, data has become digital and overwhelmingly abundant. That created both the opportunity to capture and make sense of it, but also the challenge of processing it at scale. That was the dawn of the whole “Big Data” era in the early 2010s, where technology was largely trailing behind the exploding volumes of data.
Fast forward to today, there’s been a remarkable evolution in terms of enterprises being able to tackle this challenge and becoming data-driven—even though, for industry practitioners who spend every day in the space, it sometimes feels like watching grass grow and talking about the same issues over and over. Today, many companies have, or soon will have, their “data house” in order. They often have a cloud data warehouse (like Snowflake) in place, where they can reliably and scalably store massive amounts of data. They have processes and tools in place to get the data into that cloud data warehouse and have it formatted to their needs (through what’s known at ETL or ELT). And they have ways of analyzing that data with business intelligence (BI) tools. Business intelligence is what tells you what you need to know about the present and the past.
The next big thing that has been happening over the last few years is the rise of predictive analytics in the enterprise. With the data you have about the present and the past, can you predict the near future? To continue with my cheap analogy, we as humans are constantly trying to predict throughout our day what’s about to happen next, often unconsciously, and we make thousands of decisions accordingly (that car is driving too fast, it’s going to hit me if I cross the street, so I should stay put). The enterprise needs to be able to do the same.
That's where you get into data science, machine learning, and AI. The rollout of AI in the enterprise as a core functionality that's going to be part of every single application is both very exciting and still very much in its early innings. A lot of use cases for enterprise AI so far have been focused on structured data, like churn prediction, log analysis, predictive maintenance, etc.. and demonstrated impressive ROI. The next wave is around unstructured data, like computer vision, NLP, etc. which are still nascent use cases in most enterprises.
But going back to your question, yes, that’s the big trend—to succeed, every company is going to need to be not just a software company, but also a data company. Being deeply data-driven is rapidly going from being a competitive advantage to table stakes.
Do you lean in one direction in terms of the types of businesses that you're interested in?
There are many ways of being successful as a startup in the broad data and AI market. You can be a tool or a platform that helps customers build their own data infrastructure or AI product. You can be a SasS application that embeds AI and abstracts away the complexity of it. You can be a technical dev tool, or a no-code solution. You can be open source or closed source. You can target SMBs or enterprises, horizontally or vertically. It’s a huge market.
I’m excited about all the above, and I’m an investor in anything from developer focused products like CockroachDB, Clickhouse, or Estuary, to enterprise AI platforms like Dataiku, to AI applications like Ada, Synthesia, and Hyperscience, to name a few.
Regardless of the angle, the North Star remains the same—great teams, strong products, exciting markets, and a relentless focus on the customer. That last part is particularly important for those often highly technical companies. You’re selling a solution, not a cool piece of technology. A lot of those customers are not going to care at all whether that's AI or not AI. What they're going to care about is whether it delivers value or not.
From both your experience as an investor and as an entrepreneur, how can you spot when a founder is going to be good at the work of figuring out the buyer and their intent and how to connect to them? I'm curious if you've got any tips.
Cloud, data infrastructure, and ML/AI are still very technical areas on the whole, so first and foremost I try to find founders that are very strong technically. At the same time, I tend to gravitate towards people who also have a natural commercial inclination, on top of their technical skills. I don’t expect them to have extensive experience selling and marketing or anything like that, although obviously that wouldn’t hurt, but they need to truly enjoy working with customers and solving their problems, as opposed to building fancy tech.
One simple question to ask is: "Okay, do you want to build something that's technically cool, which is great; or do you want to spend a lot of time with customers working hand-in-hand with them and sometimes babysitting them, sometimes educating them when you think they should understand better but they don't or don't have time for you?" So again, you don't need to be a born salesperson, but you need to ultimately want to make those people happy.
What is your view of the venture market and how that may be impacted by the market decline? What are some words of caution around things to be wary of? Words of encouragement?
Obviously the venture market in 2023 is drastically different from what it was in 2021-2022. Having said that, at least for now, the data and AI space feels like a bright spot, at least comparatively speaking.
There’s certainly a whole new hype cycle happening around AI right now, and a good number of deals getting done, often at valuations that are reminiscent of the height of the bull market, almost like a mini AI bubble.
Also, from a demand perspective, the general data and AI space seems to be an area where enterprises are going to continue investing even during the downturn. We’re hearing from a number of CIO-types that they view this as a 10-year investment, not a short term gain kind of thing. So there’s reason to be hopeful here.
On the other hand, if you look at the data infrastructure space, there’s more of a risk of hangover there. The huge success of the Snowflake IPO in 2020 led to a massive wave of funding in that general space, and it certainly feels really crowded now. There are tons of startups building very specialized tools that raised at high valuations and didn’t have enough time to grow their ARR before the market turned. The market feels ripe for a wave of consolidation, but probably not the kind that both founders and their VCs were hoping for.
Now of course, founders will continue building interesting companies in data infrastructure. I think there’s still plenty of opportunities, including in areas like real-time streaming which feels comparatively under-developed.
What's the renewed interest in the LLMs? I think that there are bigger models that are coming out that extend a little bit beyond what GPT-3 was doing, and it's getting more broad. There are more and more players in town, but if you have something to add?
That's exactly right. It’s been a crazy, crazy year in AI. New models, new research labs, tons of energy in the space. We’ve all seen the craze around ChatGPT, DALL-E, and Stable Diffusion. GPT-4 is rumored to be even more mind-blowing. There are plenty of open questions around those models, their accuracy, even their legality from an IP perspective. And serious concerns about whether you can build a defensible business on top of any of those platforms. But it does feel like a new era. It's just exciting.
How do you recommend finding early customers?
It’s all about personal connection and networking in the early days. Talk to your cousin or your best friend from school or whoever you met somebody in this cohort or whoever you can. Typically, the first customer is not a completely random person. Sometimes you can meet them at Data Driven NYC or any of those things. People have met customers, recruits, and even cofounders, actually, at Data Driven NYC, which has been intensely rewarding for us as organizers. But typically, for somebody to take a chance on you when you have nothing requires some deep trust in your abilities, and typically deep trust in your abilities comes through either a prior experience working with you or a super warm recommendation from somebody that person trusts. So the first few are like that.
Do you think that the immaturity of data teams and lack of data workforce enterprise customers for B2B ML ops products will force companies supplying these products towards consultancy to the point of throttling any exponential growth?
Yeah, that depends on, as always, the type of companies. The usual playbook for technical B2B startups in the early days is to focus on early adopters, who tend to be technical, sophisticated people in large startups or recently public tech companies. The bear market certainly has had an impact on that category and their propensity to experiment with and buy new products, but on the whole that strategy remains tried and true.
I am just probably stating the obvious for you all, but as a young company, I would suggest deprioritizing working with big Global 2000 non-tech companies initially because you want your product to have some level of maturity before you start getting yourself into that kind of sale, and they have security and compliance requirements that your product is just not going to be ready for.
Having said that, it can completely be done and there's a long list of companies that sold to Global 2000 companies from the beginning. You just need to have a lot of patience and be willing to go through very long sell cycles and all those things. And yes, that’s indeed in that kind of scenario that you see startups become more or less a consultancy so they can be paid on services during those long sales cycles. I don’t think there’s anything wrong with delivering professional services, by the way, but yes you probably don’t want to do that to the point of becoming a slow growth business.