Is Generative AI a Catalyst for Value-Based Care?
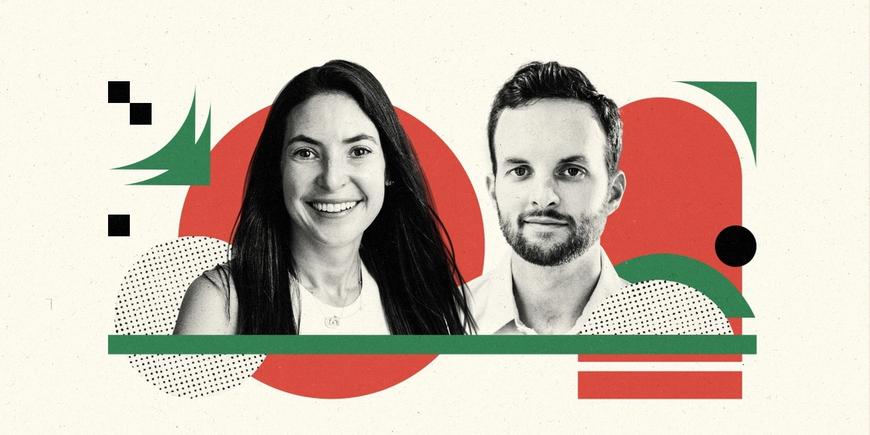
Subscribe to future editions of the Health newsletter here.
A few weeks ago, we presented in front of 50-60 health system and payor executives at Stellar Health's value-based care summit. The topic of conversation — AI and Healthcare. It’s a topic we’ve spent quite a bit of time on, but the presentation helped us crystalize our thinking and forced us to pull back and take stock of how even the past few months of GenAI progress has modified our thinking.
Is Generative AI the “aha moment” for value based care? Could it be a key ingredient to drive broader adoption and success in the value-based care world? And, if so, how does the technology start to be implemented across the healthcare sector and where does it get applied?
Before getting into the meat of this, it’s important to set the stage. During the presentation the room was full of executives, who in aggregate, control billions of dollars of spend and manage healthcare for millions of patients. They talked about the challenges of engaging patients, of too-few and burnt out providers struggling to keep their heads above water, and how the shifting healthcare landscape will continue to put economic pressure on them. Given the full plates of these execs, and the reality that many weren’t familiar with the underlying tech, we spent half the presentation walking through the core technology of LLMs, how they work, and what they are good at. The back half was spent discussing where the technology is starting to get deployed and some learnings from health systems/payers who are using it. According to Bain, roughly 75% of healthcare executives say they are interested in generative AI, yet only 6% have a strategy currently.
For many who are reading this newsletter, the learnings and themes that we discussed won’t be a surprise for you:
- First – partner, partner, partner. While most health systems have AI/ML teams, most don’t have the GenAI experience or capacity of say, OpenAI (except if you’re Oscar). When we suggest that firms partner it’s largely because none of us know (yet) which broad LLMs will win out, or whether hyper specific models will be the future (a model for ER triage, for prior auth, etc).
- Build a cross functional team across clinical, commercial and technical leadership. C-level leaders will need to lean in, given the investment and time required to be successful.
- Start small, but somewhere with a clear ROI. This is more likely to be a non-diagnostic, high volume and low risk activity; on the administrative side, we’ve seen some organizations start with prior authorization or RCM activities. Looking at a16z’s “jobs to be done” a lot of this falls into the bottom right hand quadrant.
But, if you do all of this well, how should we introduce portions of GenAI into different value-based care workstreams? Which workstreams should we start with?
As we all know, the premise of value-based care is to reward healthcare providers for improving healthcare outcomes, not just increasing volumes. We know this is massively complex (operational, financial, and technological implications) and we’ve been at this transition to VBC for a while. A core part of this transition and a driver of a long-term reduction in medical loss ratio centers around care management. As we think about the future of value based careI, we are particularly interested in how GenAI can be leveraged to augment and enhance different care management programs across the country.
Care management is a team-based, patient-centered strategy that is designed to assist patients and their support systems in managing medical and chronic conditions more effectively. The team often includes care managers, nurses, social workers, community health workers, and physicians. At a foundational level, it is organized around the concept that appropriate interventions for individuals will reduce health risks and decrease total cost of care in value-based payment structures. On the cost side, this is often seen in a reduction of ER visits and unnecessary hospitalizations for patients.
Generally, there are three key strategies for care management programs: (1) identifying population(s) with modifiable risks, generally through different risk-stratification exercises; (2) aligning care management services to the needs of the population(s); and (3) identifying appropriate personnel to deliver said services. More tactically, this is about getting in touch with patients, interacting with them in a personalized way to help them stay on a specific care plan, and ensuring patients are getting access to the appropriate resources(medical, community, and social). When this happens well, care is more personalized, patients are healthier, and healthcare costs are reduced.
Today, Generative AI, according to ChatGPT, is particularly good at natural language generation, text summarization, text-based chatbots, and language translation. We’ve seen absolutely amazing implementations of this technology being used in non-healthcare settings. So how could it be applied to care management and why does it matter?
1. Personalized patient outreach and engagement: After patients have been identified, getting in touch with them is just HARD. We’ve both seen this often takes twice as long and costs twice as much as teams initially think. Today, strategies are a one to many approach with little personalization — consider the multiple automated emails, phone calls, snail mail, and text messages sent. Personalization and communication preferences, like those we have come to expect in other industries, haven’t been adopted in healthcare at scale. Given the natural language prowess of GenAI, this is clearly very low-hanging fruit, especially as you layer in different education levels and language preferences.
2. Turbo-charging care teams: Once engaged, it’s important to align resources and turbocharge the existing care management team with a care management co-pilot. Specifically, with a core focus on enabling said team to spend more time on direct care vs indirect care activities. Looking at different studies this “indirect” bucket is estimated to take up between 10-40% of a nurse’s day. For the avoidance of doubt, we’re advocating for non-clinical communications and chatbots to start (e.g. scheduling help, medication reminders, personalized check-ins, etc). Although, we could imagine a world in the future, where there are virtual social workers, community health workers, and perhaps even nurses, who are engaging with patients — still a lot to learn here, but it’s a thought-provoking topic. Again, with the belief that all these interactions are turbo-charged by the ability to interact with patients in a more personalized and bespoke way.
3. Coordination of resources and people: A huge portion of care management is about helping patients navigate the current healthcare systems and resources available to them. Often, parts of this fall out of the scope of the daily workflows of an existing care management team and are about getting people connected to the right ancillary resources. A lot of this work falls into the social determinants of health (SDOH) bucket. If I can tell a chatbot to look-up a great chocolate chip cookie recipe online, have it order the ingredients via Instacart, and then have them delivered to my front door, shouldn’t the same bots and agents be able to do something similar for someone who is food insecure? Of course, while simultaneously layering on the ability for patients to better understand the resources they have at their disposal, properly enroll in said benefits, and then actually use them.
While many of the early implementations and “mainstream thinking” on GenAI recommend back-end focused use cases should come first, we’re taking a slightly different view and starting to make the case for GenAI-enabled care management. When you consider how this type of expansion could shift the unit economics of providing this type of service, it becomes very powerful. Instead of focusing on the 10-12% of a population who is the sickest and highest cost, what if you were able to provide the same level of service to the next 10-12% of the population? The people who are not yet as sick and as high cost. Could you preemptively bend that cost curve?
We’re also evolving our thinking about how GenAI care management programs should be implemented. For example, is EMR integration critical or a nice-to-have? We could argue some tasks might not require the longitudinal care record (e.g. scheduling support) but do need tight feedback loops with providers. Should patient and provider-facing incumbents (e.g. care delivery businesses, EMRs, etc) build these capabilities or do we think there is an opportunity for new entrants to embed themselves into these workflows? How should we think about the right metrics to track to prove the GenAI is making a difference?
Clearly there is still a lot to figure out, but fundamentally we do believe there will be opportunities to start to move towards this vision and potentially further catalyze value-based care. We’re still in the early stages of thinking about all of this and would love to hear any and all perspectives.