Frameworks from the Frontiers: 5 Things we look for when evaluating Consumer AI Companies
The questions Primary is asking as we evaluate businesses in the exciting new category of Consumer GenAI.
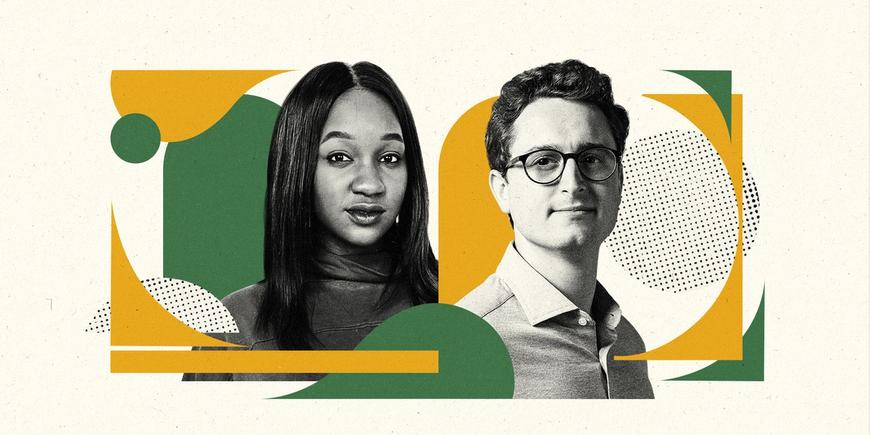
By Jason Shuman and Adetola Olatunji
New York City is home to at least 324 of 1,000 + generative AI companies across the country (and this number is rapidly growing), most of which are either early stage or have not yet raised an equity round of financing. With over $14.1 billion invested in generative AI companies nationwide as of August, we’ve seen a mad dash by Founders to reinvent consumer experiences leveraging the incredible advancements in AI.
After more than 50 calls with consumer VCs over the last few months, it has become abundantly clear that we’re in the early days of thinking regarding investor frameworks for evaluating consumer AI companies. Not only are we just beginning to realize what is actually possible with this technology, but we’re also just beginning to understand how consumer behavior will evolve as they become more familiar with the different use cases at their disposal. While some investors aren’t ready to commit to a framework or favorite categories quite yet publicly, we want to share some initial thoughts for those who might be brave enough to make the jump into starting their own companies.
Our goal is to help founders understand the opaque nature of deal analysis to support their ideation and fundraising processes. In full transparency, our framework is early and evolving every week, but here are some of our initial thoughts.
Primary’s Evaluation Framework
When looking for new consumer oriented generative AI companies, we get incredibly excited when these five elements are present:
10X consumer experiences that leverage personalization, network effects or both
Advancements in generative AI have unlocked consumer experiences that can be 10X better than what came before it or create new categories all together. Ultimately, we believe the winners leveraging this technology will build products that are either highly personalized to the user, leverage network effects, or both. They will go beyond text-first user interfaces and a wrapper on top of ChatGPT. AI will enable founders to unlock entirely new feature sets, forms of gamification, and other nudges built into the product that provide magical 10X experiences right out of the gate AND improve over time.
These highly personalized products lend themselves well to consumer subscriptions and products that can deliver better experiences with more data (where gamification can come into play), which in turn increases the odds of being able to build long-lasting customer relationships.
Text-first user interfaces and advancements in voice have incredible potential to deliver value to users in a way that incumbent products are likely to look at as secondary to the core experience they have already built. We predict that product designers are going to come up with 10X better, fundamentally different experiences that a swath of consumers will latch onto. While the long term defensibility here is questionable, the headstart opportunity is very real.
Additionally, we’re now in an era where AI agents will put concierge-level execution of tasks in the hands of consumers at scale. If prompting other horizontal products for a repetitive use case takes an unreasonable amount of time to execute the same task, a potential opportunity might be present.
Defensibility will always be top of mind for investors looking at generative AI consumer products, and will be hard for many to truly achieve. It’s cliche, but looking for companies leveraging network effects in the consumer world is critical. Network effects come in many forms, but in a world in which building a moat is increasingly harder to do, having a clear network effect that will increase over time is a critical component. Companies like Youtube, Facebook, and Roblox represented the first wave of network effect driven businesses in the early days of the internet era, while Instagram, TikTok, Doordash, Whatsapp, and others took off as part of the shift to smartphones. With all the new capabilities possible with generative AI, we think it’s safe to assume that some new network effect-driven businesses are about to be launched.
Meanwhile, some of the largest consumer outcomes historically have been subscriptions (e.g. Spotify, Netflix, etc.). In a world where generative AI businesses have very real costs to operate, having a product with a real revenue model early is not only important, but can also provide you with the ability to outspend the competition on both acquisition and R&D. To date, 90% of the top 50 consumer generative AI companies are already charging for premium subscriptions and with an average price of $21/mo. they’re taking home 2X+ what the last generation of consumer digital subscription services have been charging.
Highly technical, highly iterative teams
We have a high level of conviction that the best teams working in consumer AI will have both a strong technical founder and a strong product and/or growth oriented founder. Consumer DNA is tough to come by, but we strongly encourage technical founders who aren’t locked in on things like brand, funnel optimization and go-to-market to link up with someone complimentary to their skill set, or they’ll be at risk of getting sprinted past. Historically, we’ve been impressed by teams coming out of companies that are well known for their speed of testing and thoughtful hypothesis testing frameworks.
A Midas list VC once told Jason “first time founders think about product, second time founders think about distribution”....now is the time to be thinking about both.
Managed compute costs
The app store isn’t the only tax in town anymore. According to Insider, OpenAI is reportedly spending $700,000+ a day to operate ChatGPT. While we believe costs will come down over time, Founders absolutely need to be thinking about how to manage their compute costs, otherwise they’re going to have to raise a boatload of money to keep up with it.
Consumer subscription businesses where you can actually generate revenue to offset the compute costs offer one way to grow in a more capital efficient manner. One major key to this is the predictability of compute, which is derived from the predictability of usage. Consumer companies can be subject to compute spikes which can kill your gross margins, so thinking about more habitual vs. addictive behaviors might actually be of greater value.
At the moment, it seems like we’re also in the midst of an execution race where some companies are willing to get to scale before charging anything at all. With capital flooding into the ecosystem, it’s a tough call as to which path to take, but we anticipate that things are going to evolve on this front over time as conversion to paid users starts to play out, capital efficiency is brought more to light and we get on the other side of the funding hype cycle.
Model Selection
The other widely debated topic surrounding compute costs is the small vs. large model choice. With emergent technologies and use cases, we recognize there’s a need for flexibility as the market experiments on the business models best poised to meet consumer demand. As we think about the possibilities AI creates for 10x consumer experiences, we are excited about AI-first companies that leverage more nimble, specialized models to drive down compute costs significantly, enabling them to lower the cost passed on to consumers without sacrificing performance and capabilities. In stark contrast to the training and inference costs for Chat-GPT4 (reportedly $100M+), smaller models are substantially cheaper to run (and more environmentally-friendly), more adaptable to emergent use cases, and less time-intensive to train. On the other hand, many experts will argue that smaller models may potentially be less effective and making them better for specific use cases might be more challenging than many think…this is one topic we’re keeping a close eye on.
Because the differentiation for these startups potentially lies in how they leverage their customized datasets, our questions for founders building in this space include:
- Do you have a proprietary data set?
- What is the reason for you to build your own model?
- If you launch by leveraging external APIs, what will you want to learn/accomplish before building your own model? What are the tactical steps to doing that and who will you need to have on your team to accomplish it?
- How do you plan to acquire consumers? What have you tested previously?
- At what stage of the consumer experience do you begin monetizing a customer’s desire to continue leveraging or contributing to the personalized dataset?
- What is the time-to-value for the consumer? How delightful is that experience?
For AI-native companies leveraging larger models and / or publicly sourced datasets, differentiation is even harder to come by due to the data and distribution advantages inherent to incumbents. That being said, we’ve heard consistently from experts that generative AI applications in this category are best positioned to make headway with consumers when focused on a finite set of consumer needs as the time-to-market is usually faster and less costly. As a result, in order to get excited about a venture scale outcome we’d really like to see businesses here with distribution advantages or network effects (e.g. OpenAIs plug-in ecosystem).
Channel-market fit
In some ways, channel-market fit is like going back to the era of “As Seen on TV” where people are just wowed by the results leading not only to purchase, but also high word-of-mouth marketing.
Speed to delight or time-to-value
Products that can easily show off their magical consumer experience on channels like TikTok and Meta, or within seconds of opening your phone up for a friend, have seen a surge in demand unlike anything we’ve personally seen over the last decade. Organic growth for most consumer companies over the last five years has been incredibly challenging, but with the advancements of AI and a new user experience, many of the organic growth numbers have been eye-popping with the majority of the top 50 consumer AI companies having spent no money on paid acquisition according to SimilarWeb. As such, we’re looking for products for which consumers can appreciate the value of in < 15 seconds, as it unlocks channels of reach and potential virality that can lead to significantly healthier businesses over time.
Strong retention mechanisms and structural advantages
Products and services that users are highly likely to be using multiple times a week if not multiple times a day are the preference. In every consumer pitch meeting we walk out of, we ask ourselves: What is the probability a user will still be using this regularly in three years and why? The easier it is to answer this question with confidence that the probability is high, the easier it is for us to make an early-stage bet.
Building a deep relationship with a consumer that is rooted in frequent and deep engagement leads to strong results. Structurally, some markets and products are better than others. Similarly, when paying for products we also look for markets that have structurally sticky relationships whereby consumers are likely to be paying for the product in a few years time as well.
Conclusion
While the technology is improving at an unimaginable pace, many of the core principles for evaluating consumer AI companies leverage the same principles around any digital product and include the importance of strong teams, durable retention mechanisms and delightful, low-friction experiences for the consumer. Approaches to maximize and maintain defensibility in your product will need to remain flexible as the complexity and size of the models leveraged by founders continue to evolve. Ultimately, having a strong perspective on how you’re using external and proprietary data, how you’re attracting and maintaining initial customers, and how you create a pleasing UI/UX will set you apart from the crowd in the current age.
If you’re working on a consumer AI startup we’d love to hear from you! Follow us on X @JasonRShuman and @adetolatweets. Our DMs are open!
Thank you to the investors and Founders who helped inform our thinking, including Nikhil Basu Trivedi at Footwork, Sarah Hinkfuss at Bain Capital Ventures, Jon Bickoff at Personal.ai, Cliff Weitzman at Speechify, Ann Bordetsky at NEA, Lia Zhang at Makers Fund, Heston Berkman at Banter, Elizabeth Weil at Scribble VC, Meera Clark at Redpoint, Claire Zau at GSV, Kate Bradley Chernis at Lately.ai, Ollie Forsyth at Antler, Rex Woodbury at Daybreak and more.