Navigating the Hype and Realities of Bringing Generative AI to Financial Services
Executive appetite is real but it's still early days. Major takeaways from a tactical conversation with a group of industry experts on what’s real and what’s still to come.
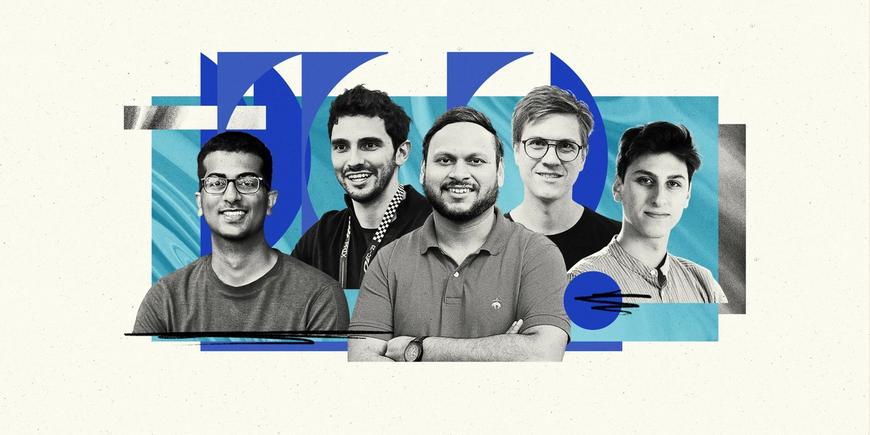
While generative AI continues to dominate the imaginations of consumers, executives, and investors alike, there are very real barriers to application and adoption in highly regulated and more conservative industries like financial services. A couple weeks ago, I sat down with five experts for a live discussion on where we are on the adoption curve of implementing Generative AI in financial services:
- Rahul Sengottuvelu (Head of Applied AI Platform, Ramp)
- Alexander Rubin (Head of Code, Scale AI)
- Pahal Patangia (Head of Developer Relations, Fintech, NVIDIA)
- Erik Bernhardsson (Co-founder & CEO, Modal Labs)
- Eugen Alpeza (AI Platform Lead, Palantir Technologies)
Across a wide ranging discussion that covered infrastructure needs, accessibility to training data, and which use cases could be tied to true ROI – four core takeaways emerged:
- Financial services is not the laggard (for once!) – Large quantities of unstructured data and the sheer volume of manual workflows makes financial services a great candidate for applications of Generative AI.
- Data curation is a critical bottleneck – Getting access to the right training data that is accurately labeled for fine-tuning models requires significant upfront investment and is a major barrier today.
- Early infrastructure decisions will determine scalability – Early decisions on about which parts of the AI stack will be owned in house AND how the AI stack will interface with the existing tech stack will have large downstream implications
- Transformative ROI will come as use cases evolve – Operational efficiencies are the obvious areas to leverage large language models (LLMs), but more imaginative use cases have yet to emerge.
Financial services is not the laggard (for once!)
Financial services companies have always been “lagging adopters” of new tech, and there are clear structural reasons (regulatory, uptime requirements, aging tech stack) why. For example, we’re decades into the shift to cloud, and many banks are only now starting to get comfortable shifting major workloads to public cloud instances.
It may be a bit different this time around. Traditional financial services companies are actively exploring ways to leverage LLM technology. “What I’ve seen is that financial services is one of the most innovative industries for gen AI,” Alexander (Scale AI) noted. “Across the enterprise, we get the most interest from financial services companies. Because they have pretty sophisticated tech teams, they’re much more willing to build out the full-stack application and can do that quickly.”
There are two main underlying drivers for this shortened adoption cycle.
Sheer quantities of unstructured data
LLMs are particularly good at taking unstructured data and turning it into structured data. Historically, the financial services industry has been heavily dependent on structured data leading to many inputs and dimensions getting lost. Now, as Pahal (NVIDIA) pointed out, “the business of arriving at insights has hugely accelerated in financial services thanks to the advent of large language models.”
Magnitude of potential efficiency gains
In banking, 20%+ of annual budgets are spent across areas that can see massive efficiency gains from gen AI automation. It’s not surprising then that, as Pahal (NVIDIA) noted, the “recent pace of advancement in gen AI is inducing a sense of urgency into executive stakeholders to capture value and gain competitive edge.”
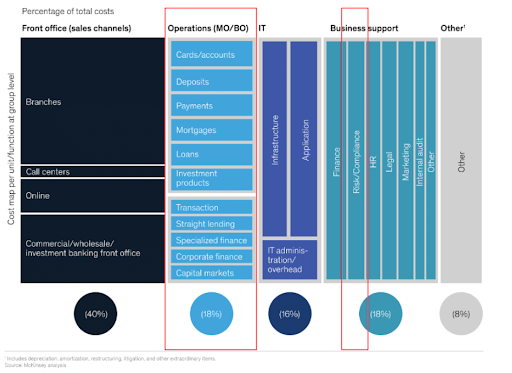
Data curation is a critical bottleneck
While execs may be salivating over the promise of LLMs and Gen AI, the reality today is that most applications still haven’t graduated beyond early exploration and POCs. Many industry players sit on a treasure trove of data, but it’s not as simple as just plugging it into an LLM and pressing “play.” Some of the hurdles include:
- Data access and availability
- “What you often need is human annotators to actually transform the data into a format that you can actually leverage for a use case.” - Alexander (Scale AI)
- “The data you have, you may not be able to use directly because you might be bound by contracts and commitments you’ve made to people.” - Rahul (Ramp)
- Data curation and quality control
- “Data curation is super important, particularly in industries that are heavily regulated and have highly sensitive information – one has to think on what their methods are to deal with PII data and clean swathes of information to ensure data quality?” - Pahal (NVIDIA)
- Data compatibility and usage
- “When data schemas evolved, they were built for non-LLMs or for humans or other systems to use and LLMs tend to think in a different way.” - Rahul (Ramp)
- “On the retrieval or embedding side, what it comes down to is actually figuring out ways to embed the data to have really high accuracy for a lot of use cases. So you have ML or product engineers figuring out novel ways to actually embed the data and how to connect different embeddings.” - Alexander (Scale AI)
Despite the challenges, Eugen (Palantir) offered a somewhat different view suggesting that, rather than investing in annotation for the sole purpose of feeding models, there’s a way to create strong feedback loops with the humans that are already doing this work day in day out. He called out that “It's kind of like a Tesla versus Waymo approach where annotation is, "I'm going to have some cars drive for no purpose other than to collect and label data," versus Tesla is much more like, "We're just going to let people use it and learn from the live operations. The platform that you're using to do the day-in, day-out work should be able to learn on its own versus having to force people to go and annotate somewhere separately.”
Early infrastructure decisions will determine scalability
On the question of whether to leverage external foundational model providers or to build and own the underlying infrastructure in house, the resounding consensus favored the latter largely due to data privacy concerns.
At Ramp, desire to vertically integrate led them to invest in building all the critical infrastructure internally: “Apart from privacy benefits, running our own inference and finetuning allows us to optimize cost and latency, and integrate models deeply with our features." For more advanced use cases, Ramp also turns to third-party providers like OpenAI and Anthropic. Rahul adds, "It’s essential to have strong contracts in place that explicitly prevent providers from using our data to train models."
Beyond the infrastructure to manage and run LLMs internally, there also needs to be a significant amount of connective tissue built that allows these models to interface with and be deeply integrated into the existing tech stack. As Eugen (Palantir) pointed out: “There's a whole set of infrastructure you need, especially as a large company, to link that with all of the rest of the infrastructure that you've built up over the last 50 years. So yeah, I might have something that will write a nice email to the customer, but you need to manage how that gets data in and then how AI can actually go and trigger an action in your CRM. So a lot of the infrastructure that needs to be built is the layer between the LLMs and all of the rest of the Frankenstein monster that you've built up over a lot of years.”
Transformative ROI will come as use cases evolve
There’s no doubt that we’re still early in identifying the best ways to leverage LLMs in fintech and financial services. While the panel was able to identify many of the obvious use cases where generative AI can immediately drive value (e.g. data extraction, vertical-specific customer support, information synthesis and insight extraction from documents, automations for certain parts of manual workflows), all agreed that the ROI and impact on these sorts of use cases is yet to be proven.
“Today, people are still focused on cost reduction,” Erik (Modal) mentioned. “That's a very uncreative way to look at new technology. Previous waves of ground-shaking technologies like the Internet or mobile phones weren’t invented to save money. They enabled new things.”
What could that look like?
Rahul (Ramp) has some ideas: “There's no text field needs to be left blank. AI could proactively suggest content based on your current task. For example, it might automatically populate a memo field, easing the user's workload. We value efficiency to the extent that ideally, users wouldn't even need to actively engage with the product; they could interact through their phones or SMS, or we could handle tasks seamlessly in the background without any intervention.”
In summary – it’s expensive, it’s early, and there’s many data and infrastructure roadblocks that still need to be ironed out but there’s a real appetite to invest behind Generative AI. It’s clear that we’ve really only just started to imagine how transformative Generative AI can be for financial services.
If you have ideas for what that could look like or are working on something in the space – we’d love to hear from you.
A huge thank you again to our panelists for joining us for a dynamic conversation and to Ramp for hosting us at the new HQ. All quotes have been lightly edited for readability but are otherwise presented verbatim.