Simplifying the Supply Chain System’s 4 Billion+ Paper Contracts
The CEO of Loop on how artificial intelligence can tackle invoice paperwork volume and complexity in the freight industry.
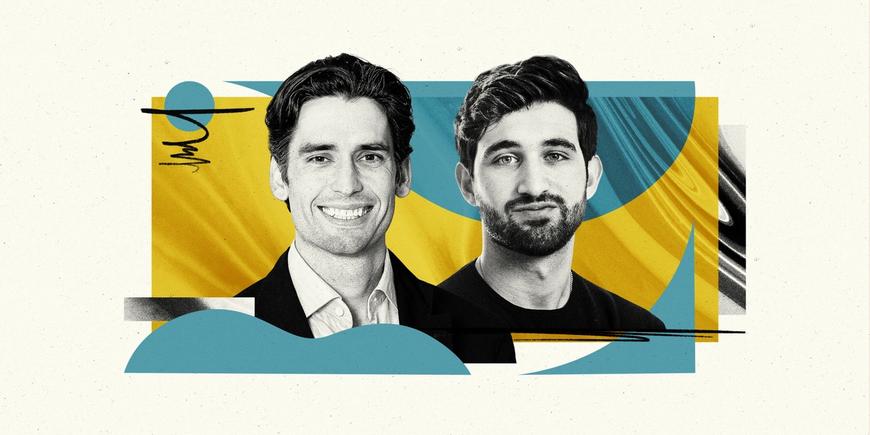
Freight invoices are wildly complex and riddled with unpredictable charges that are painstakingly slow to audit. Enter Loop, a platform created by ex-Uber and Flexport engineers promising to unlock profit trapped in the supply chain through a freight invoice audit and payments platform. Since 2021, Loop has leveraged the power of AI to revolutionize traditional supply chain invoice processes, helping companies unlock working capital and improve labor efficiency. With a $35 million Series B announced late last year, the team has been accelerating on that mission.
I sat down with Loop’s CEO Matt McKinney to discuss the transformative power of AI in modernizing supply chain operations and the company's vision for the future.
Can you provide some background on how you started Loop and what it does?
Loop was started to solve the problem of invoice reconciliation in the supply chain industry. This was a big problem when I worked at Uber Freight, but it wasn’t unique. We learned that almost every company around the supply chain faced this issue. Loop aims to solve this by addressing the overwhelming paperwork volume and the complexity of contracts.
Bloomberg reported that 4 billion pieces of paper are circulating in global trade daily, illustrating the massive scale of the problem. Contracts can also span hundreds of pages, requiring deep expertise to interpret and apply effectively. When coupled with millions of transactions, it creates a reconciliation nightmare.
Loop aims to unlock working capital and liquidity by addressing this friction, which ultimately leads to profit for companies. Whether it's benefiting shareholders or offering lower prices to consumers, our focus is on delivering tangible value to the entire industry.
Who are Loop's core customers today, and how do they view the value proposition?
Our core customers are companies involved in shipping goods, including manufacturers, distributors, retailers, and logistics service providers (LSPs) like brokers or managed transportation providers. These companies are automating previously manual workflows, which often involve large teams processing invoices daily.
For LSPs, the value proposition lies in unlocking working capital and improving labor efficiency. Consider a scenario where a 3PL has dozens or even hundreds of staff manually processing invoices, leading to significant time and capital investment. By transitioning to automation, they not only reduce manual touchpoints but also streamline operations, reallocating resources to strategic tasks like carrier selection or supplier optimization.
On the shipper side, the benefits are clear: increased automation leads to substantial cost savings and efficiency gains. By automating invoice processing, companies can redirect manpower to more value-added activities, such as negotiating better terms with suppliers or capitalizing on early payment discounts. This transformation enhances operational efficiency and empowers businesses to leverage data insights for further optimization.
How is Loop leveraging AI today, and how has that changed since the company's inception?
It's interesting to reflect on the evolution of AI in data processing. Back when 'The Attention is All You Need' paper was released, it signaled a significant shift in the landscape. I was working at Uber with my Cofounder and we knew this would change how companies process data and handle manual tasks. We didn’t think it would happen quickly, but we knew something was coming and that conventional machine learning would no longer be as valuable for these tasks. Honestly, if we hadn't seen the AI transformation coming, I don't think we would've started Loop.
It's wild how generative AI can make sense of all that messy, unstructured data. That's why we came up with 'Contextual AI' in 2022—it's like blending conventional machine learning with a human touch, giving you accuracy like a human but with the speed of a machine.
Our whole idea was to use AI to clean up, organize, and unify data, making transactions smoother. It's not just about documents either; even electronic data like EDI files can be a headache. Companies often don't stick to standard formats, throwing a PO number where it's supposed to be a destination address. A rigid machine gets confused, but with generative AI, it's like, 'Of course, that's a purchase order.' This approach still holds today, maybe even more so than it did three years ago.
Beyond just sorting out messy invoices, how else has AI expanded in your product? How has this impacted your users?
AI has evolved in our product to address workflow and fraud detection. In financial operations, like the freight market, fraud is a concern. We've developed a fraud detection system leveraging generative AI, complementing conventional AI.
Another area is automating tasks like assigning charge types to account codes and resolving exceptions within workflows. This approach optimizes workflow automation while maintaining human oversight for tasks requiring judgment. It's about leveraging AI for what it does best, while humans handle the rest.
How do you envision the future of AI in the logistics and shipping industry?
At this rate, the supply chain will skip over the digital transformation and go right into the AI transformation. You had Supply Chain 1.0 with paper, Supply Chain 2.0 with on-prem and cloud, and now companies are shifting to 3.0, integrating AI into their operations. But where we're truly headed is Supply Chain 4.0, where AI becomes the lens through which we view supply chain functions.
Imagine a complete overhaul, with AI-driven agents handling tasks like carrier calls, appointment bookings, and procurement optimization. It's a whole new way of thinking about supply chain management, with AI at its core.
How do you perceive the future of service companies compared to the adoption of direct technology and AI products by end customers?
Look, the end customer isn't concerned about the intricacies of bill auditing and payment processes. They prioritize speed, accuracy, and cost. AI delivers on all three fronts, becoming a competitive advantage.
Legacy service providers face constraints due to past decisions, making it difficult to transition to an AI-native approach. It's the innovators, like Loop, born in the AI-native era, that have a significant advantage. With ample data, esteemed customers, and capital, these companies are poised to create substantial value for end customers.
This marks just the beginning of the potential value AI can generate. With a robust platform facilitating data collection, labeling, and AI training, launching new applications becomes much more accessible compared to starting from scratch. So, in my view, it's definitely a "winner takes most" or even a "winner takes all" scenario in this market.
What use cases did you think would work for Loop but didn't, and what did you learn from that discovery process?
Our core focus has been on auditing because that's essential for reconciling invoices. To perform this audit effectively, we needed extensive data. So, we began by figuring out how to integrate invoices, contracts, and other documents into our system. But making sense of this vast amount of data was tough.
Initially, there was a prevailing notion that AI could solve all these problems. We realized that wasn’t true and considered partnering with a data labeling company. In the end, we decided to build our own system to ingest and label data at scale for higher quality. This decision significantly improved our automation rates from 60 to 90 percent.
Now, we have amassed the largest dataset of quality-labeled logistics data. This dataset has become a cornerstone in developing logistics AI, giving Loop a competitive advantage. By leveraging our platform, our customers can acquire higher-quality data more rapidly and accurately.
This journey highlighted the importance of investing in high-quality data labeling, challenging the misconception that AI could solve all problems independently. I'm glad we recognized this early on and made the necessary course corrections.
When you talk about reimagining the supply chain with AI at its core, do you envision a complete overhaul of the entire function, or do you see AI simply replacing human tasks with AI agents?
I like to think that we're not just replicating human tasks with AI, like humanoids at Tesla building cars. Simply transforming people into AI agents doing the same tasks may solve some issues, but it's not the ultimate solution. We need to explore new approaches rather than mimicking old processes. I envision a fundamental rethink of how the supply chain operates, starting from first principles. That's the way to truly transform how things get done.
What has been the biggest challenge you faced with Loop, and how did you overcome it?
One of the biggest challenges I've encountered, and believe me, there have been many, is finding and retaining top talent. It's the kind of challenge that keeps things exciting but also builds resilience.
At Loop, we're not just looking for skilled individuals; we want people who truly grasp the intricacies of our industry. This has led to months-long recruitment efforts where it felt like we'd never find the right fit. However, we've never compromised on our talent standards, even when it seemed easier to do so. Time and again, we've seen that patience pays off. While it may hurt the company in the short term, in the long run, it's the only path to success. So, finding and retaining top talent remains an ongoing challenge for us at Loop, but it's one that only makes us stronger.